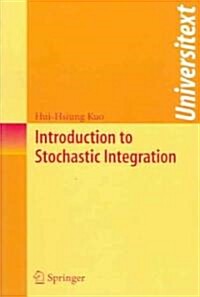
Introduction to Stochastic Integration
- 발행사항
- New York, NY : Springer, 2005
- 형태사항
- xiii, 278 p. ; 24cm
- 총서사항
- Universitext
- 서지주기
- Includes bibliographical references (p. [267]-270) and index
소장정보
위치 | 등록번호 | 청구기호 / 출력 | 상태 | 반납예정일 |
---|---|---|---|---|
이용 가능 (1) | ||||
자료실 | E206147 | 대출가능 | - |
- 등록번호
- E206147
- 상태/반납예정일
- 대출가능
- -
- 위치/청구기호(출력)
- 자료실
책 소개
Also called Ito calculus, the theory of stochastic integration has applications in virtually every scientific area involving random functions. This introductory textbook provides a concise introduction to the Ito calculus.
From the reviews:
"Introduction to Stochastic Integration is exactly what the title says. I would maybe just add a ‘friendly’ introduction because of the clear presentation and flow of the contents." --THE MATHEMATICAL SCIENCES DIGITAL LIBRARY
In the Leibniz?Newton calculus, one learns the di?erentiation and integration of deterministic functions. A basic theorem in di?erentiation is the chain rule, which gives the derivative of a composite of two di?erentiable functions. The chain rule, when written in an inde?nite integral form, yields the method of substitution. In advanced calculus, the Riemann?Stieltjes integral is de?ned through the same procedure of “partition-evaluation-summation-limit” as in the Riemann integral. In dealing with random functions such as functions of a Brownian motion, the chain rule for the Leibniz?Newton calculus breaks down. A Brownian motionmovessorapidlyandirregularlythatalmostallofitssamplepathsare nowhere di?erentiable. Thus we cannot di?erentiate functions of a Brownian motion in the same way as in the Leibniz?Newton calculus. In 1944 Kiyosi It? o published the celebrated paper “Stochastic Integral” in the Proceedings of the Imperial Academy (Tokyo). It was the beginning of the It? o calculus, the counterpart of the Leibniz?Newton calculus for random functions. In this six-page paper, It? o introduced the stochastic integral and a formula, known since then as It? o’s formula. The It? o formula is the chain rule for the It?ocalculus.Butitcannotbe expressed as in the Leibniz?Newton calculus in terms of derivatives, since a Brownian motion path is nowhere di?erentiable. The It? o formula can be interpreted only in the integral form. Moreover, there is an additional term in the formula, called the It? o correction term, resulting from the nonzero quadratic variation of a Brownian motion.
New feature
The theory of stochastic integration, also called the Ito calculus, has a large spectrum of applications in virtually every scientific area involving random functions, but it can be a very difficult subject for people without much mathematical background. The Ito calculus was originally motivated by the construction of Markov diffusion processes from infinitesimal generators. Previously, the construction of such processes required several steps, whereas Ito constructed these diffusion processes directly in a single step as the solutions of stochastic integral equations associated with the infinitesimal generators. Moreover, the properties of these diffusion processes can be derived from the stochastic integral equations and the Ito formula. This introductory textbook on stochastic integration provides a concise introduction to the Ito calculus, and covers the following topics:
* Constructions of Brownian motion;
* Stochastic integrals for Brownian motion and martingales;
* The Ito formula;
* Multiple Wiener-Ito integrals;
* Stochastic differential equations;
* Applications to finance, filtering theory, and electric circuits.
The reader should have a background in advanced calculus and elementary probability theory, as well as a basic knowledge of measure theory and Hilbert spaces. Each chapter ends with a variety of exercises designed to help the reader further understand the material.
Hui-Hsiung Kuo is the Nicholson Professor of Mathematics at Louisiana State University. He has delivered lectures on stochastic integration at Louisiana State University, Cheng Kung University, Meijo University, and University of Rome "Tor Vergata," among others. He is also the author of Gaussian Measures in Banach Spaces (Springer 1975), and White Noise Distribution Theory (CRC Press 1996), and a memoir of his childhood growing up in Taiwan, An Arrow Shot into the Sun (Abridge Books 2004).