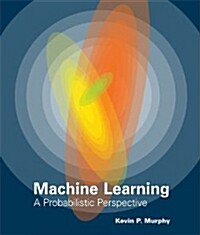
Machine Learning: A Probabilistic Perspective
- 발행사항
- Cambridge, MA : The MIT Press, 2012
- 형태사항
- xxix, 1067 p. : ill. (some col.) ; 24 cm
- 서지주기
- Includes bibliographical references (p. [1015]-1045) and indexes
소장정보
위치 | 등록번호 | 청구기호 / 출력 | 상태 | 반납예정일 |
---|---|---|---|---|
지금 이용 불가 (1) | ||||
자료실 | E207094 | 대출중 | 2025.05.19 |
- 등록번호
- E207094
- 상태/반납예정일
- 대출중
- 2025.05.19
- 위치/청구기호(출력)
- 자료실
책 소개
Today's Web-enabled deluge of electronic data calls for automated methods of data analysis. Machine learning provides these, developing methods that can automatically detect patterns in data and then use the uncovered patterns to predict future data. This textbook offers a comprehensive and self-contained introduction to the field of machine learning, based on a unified, probabilistic approach.
The coverage combines breadth and depth, offering necessary background material on such topics as probability, optimization, and linear algebra as well as discussion of recent developments in the field, including conditional random fields, L1 regularization, and deep learning. The book is written in an informal, accessible style, complete with pseudo-code for the most important algorithms. All topics are copiously illustrated with color images and worked examples drawn from such application domains as biology, text processing, computer vision, and robotics. Rather than providing a cookbook of different heuristic methods, the book stresses a principled model-based approach, often using the language of graphical models to specify models in a concise and intuitive way. Almost all the models described have been implemented in a MATLAB software package—PMTK (probabilistic modeling toolkit)—that is freely available online. The book is suitable for upper-level undergraduates with an introductory-level college math background and beginning graduate students.
Reviews
This comprehensive book should be of great interest to learners and practitioners in the field of machine learning.—British Computer Society—About the Author
Kevin P. Murphy is a Senior Staff Research Scientist at Google Research.