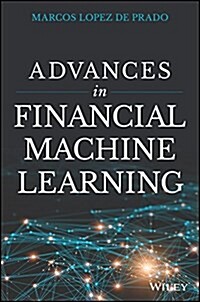
Advances in Financial Machine Learning
- 발행사항
- New Jersey : Wiley, 2018
- 형태사항
- xxi, 366 p. : illustrations ; 24cm
- 서지주기
- Include reference amd index
소장정보
위치 | 등록번호 | 청구기호 / 출력 | 상태 | 반납예정일 |
---|---|---|---|---|
이용 가능 (1) | ||||
자료실 | E207190 | 대출가능 | - |
- 등록번호
- E207190
- 상태/반납예정일
- 대출가능
- -
- 위치/청구기호(출력)
- 자료실
책 소개
Machine learning (ML) is changing virtually every aspect of our lives. Today ML algorithms accomplish tasks that until recently only expert humans could perform. As it relates to finance, this is the most exciting time to adopt a disruptive technology that will transform how everyone invests for generations. Readers will learn how to structure Big data in a way that is amenable to ML algorithms; how to conduct research with ML algorithms on that data; how to use supercomputing methods; how to backtest your discoveries while avoiding false positives. The book addresses real-life problems faced by practitioners on a daily basis, and explains scientifically sound solutions using math, supported by code and examples. Readers become active users who can test the proposed solutions in their particular setting. Written by a recognized expert and portfolio manager, this book will equip investment professionals with the groundbreaking tools needed to succeed in modern finance.
New feature
Today's machine learning (ML) algorithms have conquered the major strategy games, and are routinely used to execute tasks once only possible by a limited group of experts. Over the next few years, ML algorithms will transform finance beyond anything we know today. Advances in Financial Machine Learning was written for the investment professionals and data scientists at the forefront of this evolution.
This one-of-a-kind, practical guidebook is your go-to resource of authoritative insight into using advanced ML solutions to overcome real-world investment problems. It demystifies the entire subject and unveils cutting-edge ML techniques specific to investing. With step-by-step clarity and purpose, it quickly brings you up to speed on fully proven approaches to data analysis, model research, and discovery evaluation. Then, it shines a light on the nuanced details behind innovative ways to extract informative features from financial data. To streamline implementation, it gives you valuable recipes for high-performance computing systems optimized to handle this type of financial data analysis.
Advances in Financial Machine Learning crosses the proverbial divide that separates academia and the industry. It does not advocate a theory merely because of its mathematical beauty, and it does not propose a solution just because it appears to work. The author transmits the kind of knowledge that only comes from experience, formalized in a rigorous manner.
This turnkey guide is designed to be immediately useful to the practitioner by featuring code snippets and hands-on exercises that facilitate the quick absorption and application of best practices in the real world.
Stop guessing and profit off data by:
- Tackling today's most challenging aspects of applying ML algorithms to financial strategies, including backtest overfitting
- Using improved tactics to structure financial data so it produces better outcomes with ML algorithms
- Conducting superior research with ML algorithms as well as accurately validating the solutions you discover
- Learning the tricks of the trade from one of the largest ML investment managers
Put yourself ahead of tomorrow's competition today with Advances in Financial Machine Learning.
Praise for ADVANCES in FINANCIAL MACHINE LEARNING
"Dr. López de Prado has written the first comprehensive book describing the application of modern ML to financial modeling. The book blends the latest technological developments in ML with critical life lessons learned from the author's decades of financial experience in leading academic and industrial institutions. I highly recommend this exciting book to both prospective students of financial ML and the professors and supervisors who teach and guide them." PROF. PETER CARR, Chair of the Finance and Risk Engineering Department, NYU Tandon School of Engineering
"Financial problems require very distinct machine learning solutions. Dr. López de Prado's book is the first one to characterize what makes standard machine learning tools fail when applied to the field of finance, and the first one to provide practical solutions to unique challenges faced by asset managers. Everyone who wants to understand the future of finance should read this book." PROF. FRANK FABOZZI, EDHEC Business School; Editor of The Journal of Portfolio Management
"Marcos has assembled in one place an invaluable set of lessons and techniques for practitioners seeking to deploy machine learning methods in finance. Marcos's insightful book is laden with useful advice to help keep a curious practitioner from going down any number of blind alleys, or shooting oneself in the foot." ROSS GARON, Head of Cubist Systematic Strategies; Managing Director, Point72 Asset Management
"The first wave of quantitative innovation in finance was led by Markowitz optimization. Machine learning is the second wave and it will touch every aspect of finance. López de Prado's Advances in Financial Machine Learning is essential for readers who want to be ahead of the technology rather than being replaced by it." PROF. CAMPBELL HARVEY, Duke University; Former President of the American Finance Association
"The author's academic and professional first-rate credentials shine through the pages of this book indeed, I could think of few, if any, authors better suited to explaining both the theoretical and the practical aspects of this new and (for most) unfamiliar subject. Destined to become a classic in this rapidly burgeoning field." PROF. RICCARDO REBONATO, EDHEC Business School; Former Global Head of Rates and FX Analytics at PIMCO