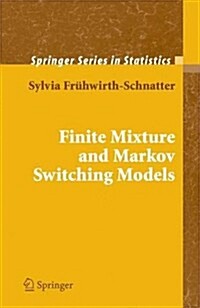
Finite Mixture and Markov Switching Models
- 발행사항
- New York : Springer, 2006
- 형태사항
- xix, 492p. ; 25cm
- 서지주기
- 색인수록
소장정보
위치 | 등록번호 | 청구기호 / 출력 | 상태 | 반납예정일 |
---|---|---|---|---|
이용 가능 (1) | ||||
자료실 | E203647 | 대출가능 | - |
- 등록번호
- E203647
- 상태/반납예정일
- 대출가능
- -
- 위치/청구기호(출력)
- 자료실
책 소개
The past decade has seen powerful new computational tools for modeling which combine a Bayesian approach with recent Monte simulation techniques based on Markov chains. This book is the first to offer a systematic presentation of the Bayesian perspective of finite mixture modelling. The book is designed to show finite mixture and Markov switching models are formulated, what structures they imply on the data, their potential uses, and how they are estimated. Presenting its concepts informally without sacrificing mathematical correctness, it will serve a wide readership including statisticians as well as biologists, economists, engineers, financial and market researchers.
This book is the first to offer a systematic presentation of the Bayesian perspective of finite mixture modeling, showing how finite mixture and Markov switching models are formulated, what structures they imply on the data, their potential uses, and how they are estimated.
New feature
The prominence of finite mixture modelling is greater than ever. Many important statistical topics like clustering data, outlier treatment, or dealing with unobserved heterogeneity involve finite mixture models in some way or other. The area of potential applications goes beyond simple data analysis and extends to regression analysis and to non-linear time series analysis using Markov switching models.
For more than the hundred years since Karl Pearson showed in 1894 how to estimate the five parameters of a mixture of two normal distributions using the method of moments, statistical inference for finite mixture models has been a challenge to everybody who deals with them. In the past ten years, very powerful computational tools emerged for dealing with these models which combine a Bayesian approach with recent Monte simulation techniques based on Markov chains. This book reviews these techniques and covers the most recent advances in the field, among them bridge sampling techniques and reversible jump Markov chain Monte Carlo methods.
It is the first time that the Bayesian perspective of finite mixture modelling is systematically presented in book form. It is argued that the Bayesian approach provides much insight in this context and is easily implemented in practice. Although the main focus is on Bayesian inference, the author reviews several frequentist techniques, especially selecting the number of components of a finite mixture model, and discusses some of their shortcomings compared to the Bayesian approach.
The aim of this book is to impart the finite mixture and Markov switching approach to statistical modelling to a wide-ranging community. This includes not only statisticians, but also biologists, economists, engineers, financial agents, market researcher, medical researchers or any other frequent user of statistical models. This book should help newcomers to the field to understand how finite mixture and Markov switching models are formulated, what structures they imply on the data, what they could be used for, and how they are estimated. Researchers familiar with the subject also will profit from reading this book. The presentation is rather informal without abandoning mathematical correctness. Previous notions of Bayesian inference and Monte Carlo simulation are useful but not needed.
Sylvia Fruhwirth-Schnatter is Professor of Applied Statistics and Econometrics at the Department of Applied Statistics of the Johannes Kepler University in Linz, Austria. She received her Ph.D. in mathematics from the University of Technology in Vienna in 1988. She has published in many leading journals in applied statistics and econometrics on topics such as Bayesian inference, finite mixture models, Markov switching models, state space models, and their application in marketing, economics and finance.
목차
Finite Mixture Modelling.- Statistical Inference for a Finite Mixture Model with Known Number of Components.- Practical Bayesian Inference for A Finite Mixture Model With Known Number of Components.- Statistical Inference for Finite Mixture Models Under Model Specification Uncertainty.- Computational Tools for Bayesian Inference for Finite Mixture Models Under Model Specification Uncertainty.- Finite Mixture Models With Normal Components.- Data Analysis Based on Finite Mixtures.- Finite Mixtures of Regression Models.- Finite Mixture Models with Non-Normal Components.- Finite Markov Mixture Modelling.- Statistical Inference for Markov Switching Models.- Non-Linear Time Series Analysis Based on Markov Switching Models.- Switching State Space Models.