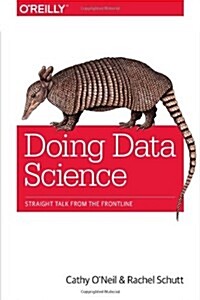
단행본
Doing Data Science: Straight Talk From the Frontline
- 판사항
- First edition
- 발행사항
- Sebastopol, CA : O'Reilly, 2013
- 형태사항
- xxiv, 375 p. : illustrations ; 23 cm
- 서지주기
- Includes index
소장정보
위치 | 등록번호 | 청구기호 / 출력 | 상태 | 반납예정일 |
---|---|---|---|---|
이용 가능 (1) | ||||
자료실 | E207072 | 대출가능 | - |
이용 가능 (1)
- 등록번호
- E207072
- 상태/반납예정일
- 대출가능
- -
- 위치/청구기호(출력)
- 자료실
책 소개
Now that answering complex and compelling questions with data can make the difference in an election or a business model, data science is an attractive discipline. But how can you learn this wide-ranging, interdisciplinary field? With this book, you’ll get material from Columbia University’s "Introduction to Data Science" class in an easy-to-follow format.Each chapter-long lecture features a guest data scientist from a prominent company such as Google, Microsoft, or eBay teaching new algorithms, methods, or models by sharing case studies and actual code they use. You’ll learn what’s involved in the lives of data scientists and be able to use the techniques they present.Guest lectures focus on topics such as:Machine learning and data mining algorithms Statistical models and methods Prediction vs. description Exploratory data analysis Communication and visualization Data processing Big data Programming Ethics Asking good questions If you’re familiar with linear algebra, probability and statistics, and have some programming experience, this book will get you started with data science.Doing Data Science is collaboration between course instructor Rachel Schutt (also employed by Google) and data science consultant Cathy O’Neil (former quantitative analyst for D.E. Shaw) who attended and blogged about the course.
목차
Preface
1. Introduction: What Is Data Science?
2. Statistical Inference, Exploratory Data Analysis, and the Data Science Process
3. Algorithms
4. Spam Filters, Naive Bayes, and Wrangling
5. Logistic Regression
6. Time Stamps and Financial Modeling
7. Extracting Meaning from Data
8. Recommendation Engines: Building a User-Facing Data Product at Scale
9. Data Visualization and Fraud Detection
10. Social Networks and Data Journalism
11. Causality
12. Epidemiology
13. Lessons Learned from Data Competitions: Data Leakage and Model Evaluation
14. Data Engineering: MapReduce, Pregel, and Hadoop
15. The Students Speak
16. Next-Generation Data Scientists, Hubris, and Ethics
Index