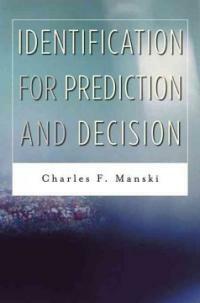
Identification for Prediction and Decision
- 발행사항
- Cambridge, Mass. : Harvard University Press, 2007
- 형태사항
- xiv, 348 p. ; 25 cm
- 서지주기
- Includes bibliographical references (p. [321]-337) and indexes
소장정보
위치 | 등록번호 | 청구기호 / 출력 | 상태 | 반납예정일 |
---|---|---|---|---|
이용 가능 (1) | ||||
자료실 | E205191 | 대출가능 | - |
- 등록번호
- E205191
- 상태/반납예정일
- 대출가능
- -
- 위치/청구기호(출력)
- 자료실
책 소개
This book is a full-scale exposition of Charles Manski's new methodology for analyzing empirical questions in the social sciences. He recommends that researchers first ask what can be learned from data alone, and then ask what can be learned when data are combined with credible weak assumptions. Inferences predicated on weak assumptions, he argues, can achieve wide consensus, while ones that require strong assumptions almost inevitably are subject to sharp disagreements.
Building on the foundation laid in the author's Identification Problems in the Social Sciences (Harvard, 1995), the book's fifteen chapters are organized in three parts. Part I studies prediction with missing or otherwise incomplete data. Part II concerns the analysis of treatment response, which aims to predict outcomes when alternative treatment rules are applied to a population. Part III studies prediction of choice behavior.
Each chapter juxtaposes developments of methodology with empirical or numerical illustrations. The book employs a simple notation and mathematical apparatus, using only basic elements of probability theory.