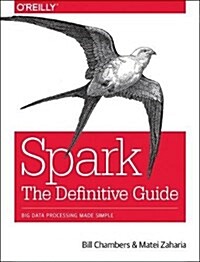
Spark: The Definitive Guide: Big Data Processing Made Simple
- 판사항
- 1st Edition
- 발행사항
- Sebastapol, CA : O'Reilly Media, 2018
- 형태사항
- xxvi, 576p. : illustrations ; 24cm
- 서지주기
- Includes index
소장정보
위치 | 등록번호 | 청구기호 / 출력 | 상태 | 반납예정일 |
---|---|---|---|---|
이용 가능 (1) | ||||
자료실 | E207405 | 대출가능 | - |
- 등록번호
- E207405
- 상태/반납예정일
- 대출가능
- -
- 위치/청구기호(출력)
- 자료실
책 소개
Learn how to use, deploy, and maintain Apache Spark with this comprehensive guide, written by the creators of this open-source cluster-computing framework. With an emphasis on improvements and new features in Spark 2.0, authors Bill Chambers and Matei Zaharia break down Spark topics into distinct sections, each with unique goals.
You’ll explore the basic operations and common functions of Spark’s structured APIs, as well as Structured Streaming, a new high-level API for building end-to-end streaming applications. Developers and system administrators will learn the fundamentals of monitoring, tuning, and debugging Spark, and explore machine learning techniques and scenarios for employing MLlib, Spark’s scalable machine learning library.
- Get a gentle overview of big data and Spark
- Learn about DataFrames, SQL, and Datasets?Spark’s core APIs?through worked examples
- Dive into Spark’s low-level APIs, RDDs, and execution of SQL and DataFrames
- Understand how Spark runs on a cluster
- Debug, monitor, and tune Spark clusters and applications
- Learn the power of Spark’s Structured Streaming and MLlib for machine learning tasks
- Explore the wider Spark ecosystem, including SparkR and Graph Analysis
- Examine Spark deployment, including coverage of Spark in the Cloud