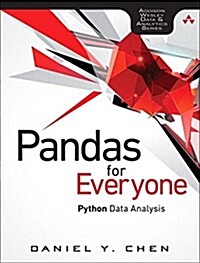
Pandas for Everyone: Python Data Analysis
- 발행사항
- Boston : Addison-Wesley Professional, 2018
- 형태사항
- xxix, 376p. : illustrations ; 24cm
- 서지주기
- Includes index
소장정보
위치 | 등록번호 | 청구기호 / 출력 | 상태 | 반납예정일 |
---|---|---|---|---|
이용 가능 (1) | ||||
자료실 | E207054 | 대출가능 | - |
- 등록번호
- E207054
- 상태/반납예정일
- 대출가능
- -
- 위치/청구기호(출력)
- 자료실
책 소개
The Hands-On, Example-Rich Introduction to Pandas Data Analysis in Python
Today, analysts must manage data characterized by extraordinary variety, velocity, and volume. Using the open source Pandas library, you can use Python to rapidly automate and perform virtually any data analysis task, no matter how large or complex. Pandas can help you ensure the veracity of your data, visualize it for effective decision-making, and reliably reproduce analyses across multiple datasets.
Pandas for Everyone brings together practical knowledge and insight for solving real problems with Pandas, even if you’re new to Python data analysis. Daniel Y. Chen introduces key concepts through simple but practical examples, incrementally building on them to solve more difficult, real-world problems.
Chen gives you a jumpstart on using Pandas with a realistic dataset and covers combining datasets, handling missing data, and structuring datasets for easier analysis and visualization. He demonstrates powerful data cleaning techniques, from basic string manipulation to applying functions simultaneously across dataframes.
Once your data is ready, Chen guides you through fitting models for prediction, clustering, inference, and exploration. He provides tips on performance and scalability, and introduces you to the wider Python data analysis ecosystem.
- Work with DataFrames and Series, and import or export data
- Create plots with matplotlib, seaborn, and pandas
- Combine datasets and handle missing data
- Reshape, tidy, and clean datasets so they’re easier to work with
- Convert data types and manipulate text strings
- Apply functions to scale data manipulations
- Aggregate, transform, and filter large datasets with groupby
- Leverage Pandas’ advanced date and time capabilities
- Fit linear models using statsmodels and scikit-learn libraries
- Use generalized linear modeling to fit models with different response variables
- Compare multiple models to select the “best”
- Regularize to overcome overfitting and improve performance
- Use clustering in unsupervised machine learning