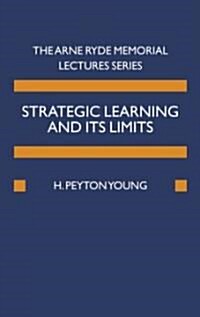
단행본
Strategic Learning and Its Limits
- 발행사항
- Oxford [England] ; New York : Oxford University Press, 2005
- 형태사항
- x, 165 p. : ill ; 23cm
- 서지주기
- Includes bibliographical references (p. [149]-158) and index
소장정보
위치 | 등록번호 | 청구기호 / 출력 | 상태 | 반납예정일 |
---|---|---|---|---|
이용 가능 (1) | ||||
자료실 | E206286 | 대출가능 | - |
이용 가능 (1)
- 등록번호
- E206286
- 상태/반납예정일
- 대출가능
- -
- 위치/청구기호(출력)
- 자료실
책 소개
In this book a distinguished economist suggests a conceptual framework for studying strategic learning, one of the key theoretical developments in current economics. He discusses the interactive learning problem; reinforcement and regret; equilibrium; conditional no-regret learning; prediction, postdiction, and calibration; fictitious play and its variants; Bayesian learning; and hypothesis testing.
In this concise book based on his Arne Ryde Lectures in 2002, Young suggests a conceptual framework for studying strategic learning and highlights theoretical developments in the area. He discusses the interactive learning problem; reinforcement and regret; equilibrium; conditional no-regret learning; prediction, postdiction, and calibration; fictitious play and its variants; Bayesian learning; and hypothesis testing. Young's framework emphasizes the amount of information required to implement different types of learning rules, criteria for evaluating their performance, and alternative notions of equilibrium to which they converge. He also stresses the limits of what can be achieved: for a given type of game and a given amount of information, there may exist no learning procedure that satisfies certain reasonable criteria of performance and convergence. In short, Young has provided a valuable primer that delineates what we know, what we would like to know, and the limits of what we can know, when we try to learn about a system that is composed of other learners.
In this concise book based on his Arne Ryde Lectures in 2002, Young suggests a conceptual framework for studying strategic learning and highlights theoretical developments in the area. He discusses the interactive learning problem; reinforcement and regret; equilibrium; conditional no-regret learning; prediction, postdiction, and calibration; fictitious play and its variants; Bayesian learning; and hypothesis testing. Young's framework emphasizes the amount of information required to implement different types of learning rules, criteria for evaluating their performance, and alternative notions of equilibrium to which they converge. He also stresses the limits of what can be achieved: for a given type of game and a given amount of information, there may exist no learning procedure that satisfies certain reasonable criteria of performance and convergence. In short, Young has provided a valuable primer that delineates what we know, what we would like to know, and the limits of what we can know, when we try to learn about a system that is composed of other learners.
목차
Contents
Preface ix
Acknowledgements x
1.The Interactive Learning Problem 1
2.Reinforcement and Regret 10
2.1.Reinforcement learning 10
2.2.Learning in stationary environments 16
2.3.Criteria of performance 18
2.4.Regret 19
2.5.Regret matching 21
2.6.Realized payoffs 22
2.7.The logic of regret matching 25
3.Equilibrium 29
3.1.Forms of equilibrium 29
3.2.Examples 32
3.3.A generalization of correlated equilibrium 34
3.4.Learning coarse correlated equilibrium 36
3.5.Concepts of convergence 39
4.Conditional NoRegret Learning 43
4.1.Conditional versus unconditional regret 43
4.2.Blackwells approachability theorem 45
4.3.Eliminating conditional regret 51
4.4.Simple rules eliminating conditional regret 54
4.5.A generalization of Blackwells theorem 57
4.6.Summary 60
5.Prediction, Postdiction, and Calibration 62
5.1.Prediction of an unknown process 62
5.2.An impossibility theorem of Oakes 64
5.3.Random forecasting rules 68
5.4.Fosters forecasting rule 72
5.5.Calibrated forecasting and correlated equilibrium 74
6.Fictitious Play and Its Variants 76
6.1.Predictive learning rules 76
6.2.Smoothed fictitious play 80
6.3.Better versus best reply 83
6.4.Finite memory and inertia 84
6.5.Convergence for weakly acyclic games 86
7.Bayesian Learning 91
7.1.The inference problem 91
7.2.An example 92
7.3.Strategies and beliefs 95
7.4.Optimality and equilibrium 99
7.5.Uncertainty and robustness 103
7.6.An impossibility theorem 106
7.7.Further implications 110
8.Hypothesis Testing 113
8.1.Cognitive learning theory 113
8.2.Cognitive learning in games 114
8.3.The structure of hypothesis testing 117
8.4.Nave hypothesis testing 119
8.5.Dynamics of hypothesis testing 121
8.6.Learning Nash equilibrium 129
8.7.Hypothesis testing: the general case 131
8.8.Models, hunches, and beliefs 137
8.9.Convergence in probability 139
8.10.Learning to predict 142
9.Conclusion 144