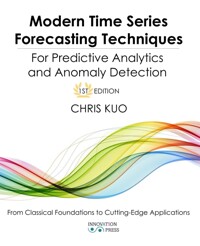
Modern Time Series Forecasting Techniques For Predictive Analytics and Anomaly Detection: From Classical Foundations to Cutting-Edge
- 판사항
- 1st edition
- 발행사항
- The innovation Press, 2024
- 형태사항
- 269 pages: illustrations; 27 cm
- 서지주기
- Includes bibliographical references and index
소장정보
위치 | 등록번호 | 청구기호 / 출력 | 상태 | 반납예정일 |
---|---|---|---|---|
지금 이용 불가 (1) | ||||
자료실 | E208436 | 대출중 | 2025.06.23 |
- 등록번호
- E208436
- 상태/반납예정일
- 대출중
- 2025.06.23
- 위치/청구기호(출력)
- 자료실
책 소개
This book organizes time series models and applications into six meticulously crafted parts. Each part equips you with the knowledge and skills to conduct time series forecasting and anomaly detection. The six parts are:
Part 1: From Prophet to NeuralProphet
Part 2: Getting Probabilistic Forecasts
Part 3: Autoregressive-based Time Series Techniques
Part 4: Tree-based Time Series Techniques
Part 5: Deep into Deep learning-based Time Series Techniques
Part 6: Transformer-based Time Series Techniques
WHY READ THIS BOOK?
In this book, you will find a wide coverage of methodologies, algorithms, and applications. Whether you’re a seasoned data scientist seeking to refine your expertise or a novice eager to embark on your analytical odyssey, this book offers a roadmap tailored to cater to diverse skill levels and objectives. You will emerge equipped with the proficiency and confidence to unravel intricate temporal patterns, harness predictive power, and unlock new horizons of insight across a myriad of domains, from finance and economics to healthcare and beyond. The writing style of this book is another selling point. Instead of taking a technique as given, this book first describes intuitions and then dives into detail. This book also gives a landscaping view from one idea to the consequent ideas. With the real-world data cases in this book, you will gain a deeper understanding of how time series techniques are applied in diverse domains.
WHAT YOU GET IN THE BOOK
Learning time series techniques comprehensively in a short period of time
A roadmap from the classical techniques to modern time series forecasting
Applying forecasting for resource planning and anomaly detection
Mastering time series Python libraries
Model interpretability
Model evaluation metrics
Hands-on example code
Cheat Sheets
목차
Preface
Introduction
Prophet for business forecasting
Tutorial I
Tutorial II
Change Point Detection in Time Series
Monte Carlo Simulation for Probabilistic Forecasting
Quantile Regression for Probabilistic Forecasting
Conformal Predictions for Probabilistic Forecasting
Conformalized Quantile Regression for Probabilistic Forecasting
Automatic ARIMA!
Time Series Data Formats Made Easy
Linear Regression for Multi-period Probabilistic Forecasting
Feature Engineering for Tree-based Time Series Models
Two Primary Strategies for Multi-period Time Series Forecasting
Tree-based XGB, LightGBM, and CatBoost Models for Multi-period Probabilistic Forecasting
The Progression of Time Series Modeling Techniques
Deep Learning-based DeepAR for Probabilistic Forecasting
Application ? Probabilistic Predictions for stock prices
From RNN to Transformer-based Time Series Models
Temporal Fusion Transformer for Interpretable Time Series Predictions
Lag-Llama for Time Series Forecasting