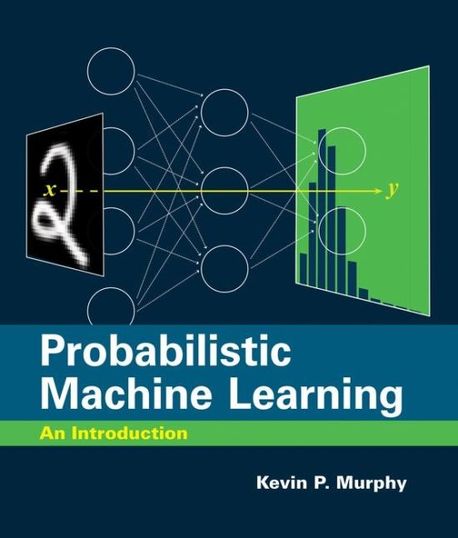
Probabilistic Machine Learning: An Introduction
- 발행사항
- MIT Press 2022
- 형태사항
- xxix, 826 pages: ill; 24 cm
- 서지주기
- Includes bibliographical references and index
소장정보
위치 | 등록번호 | 청구기호 / 출력 | 상태 | 반납예정일 |
---|---|---|---|---|
지금 이용 불가 (1) | ||||
자료실 | E208385 | 대출중 | 2025.06.11 |
- 등록번호
- E208385
- 상태/반납예정일
- 대출중
- 2025.06.11
- 위치/청구기호(출력)
- 자료실
책 소개
This book offers a detailed and up-to-date introduction to machine learning (including deep learning) through the unifying lens of probabilistic modeling and Bayesian decision theory. The book covers mathematical background (including linear algebra and optimization), basic supervised learning (including linear and logistic regression and deep neural networks), as well as more advanced topics (including transfer learning and unsupervised learning). End-of-chapter exercises allow students to apply what they have learned, and an appendix covers notation.
Probabilistic Machine Learning grew out of the author’s 2012 book, Machine Learning: A Probabilistic Perspective. More than just a simple update, this is a completely new book that reflects the dramatic developments in the field since 2012, most notably deep learning. In addition, the new book is accompanied by online Python code, using libraries such as scikit-learn, JAX, PyTorch, and Tensorflow, which can be used to reproduce nearly all the figures; this code can be run inside a web browser using cloud-based notebooks, and provides a practical complement to the theoretical topics discussed in the book. This introductory text will be followed by a sequel that covers more advanced topics, taking the same probabilistic approach.
About the Author
Kevin P. Murphy is a Research Scientist at Google in Mountain View, California, where he works on AI, machine learning, computer vision, and natural language understanding.목차
1 Introduction 1
I Foundations 29
2 Probability: Univariate Models 31
3 Probability: Multivariate Models 75
4 statistics 103
5 Decision Theory 163
6 Information Theory 199
7 Linear Algebra 221
8 Optimization 269
II Linear Models 315
9 Linear Discriminant Analysis 317
10 Logistic Regression 333
11 Linear Regression 365
12 Generalized Linear Models * 409
III Deep Neural Networks 417
13 Neural Networks for Structured Data 419
14 Neural Networks for Images 461
15 Neural Networks for Sequences 497
IV Nonparametric Models 539
16 Exemplar-based Methods 541
17 Kernel Methods * 561
18 Trees, Forests, Bagging, and Boosting 597
V Beyond Supervised Learning 619
19 Learning with Fewer Labeled Examples 621
20 Dimensionality Reduction 651
21 Clustering 709
22 Recommender Systems 735
23 Graph Embeddings * 747
A Notation 767