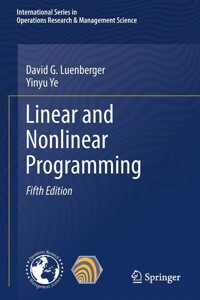
Linear and Nonlinear Programming
- 발행사항
- Cham: Springer 2022
- 형태사항
- XV, 609 pages: illustrations; 24 cm
소장정보
위치 | 등록번호 | 청구기호 / 출력 | 상태 | 반납예정일 |
---|---|---|---|---|
지금 이용 불가 (1) | ||||
자료실 | E208387 | 대출중 | 2025.06.11 |
- 등록번호
- E208387
- 상태/반납예정일
- 대출중
- 2025.06.11
- 위치/청구기호(출력)
- 자료실
책 소개
The 5th edition of this classic textbook covers the central concepts of practical optimization techniques, with an emphasis on methods that are both state-of-the-art and popular. One major insight is the connection between the purely analytical character of an optimization problem and the behavior of algorithms used to solve that problem. End-of-chapter exercises are provided for all chapters.
The material is organized into three separate parts. Part I offers a self-contained introduction to linear programming. The presentation in this part is fairly conventional, covering the main elements of the underlying theory of linear programming, many of the most effective numerical algorithms, and many of its important special applications. Part II, which is independent of Part I, covers the theory of unconstrained optimization, including both derivations of the appropriate optimality conditions and an introduction to basic algorithms. This part of the book explores the general properties of algorithms and defines various notions of convergence. In turn, Part III extends the concepts developed in the second part to constrained optimization problems. Except for a few isolated sections, this part is also independent of Part I. As such, Parts II and III can easily be used without reading Part I and, in fact, the book has been used in this way at many universities.
New to this edition are popular topics in data science and machine learning, such as the Markov Decision Process, Farkas’ lemma, convergence speed analysis, duality theories and applications, various first-order methods, stochastic gradient method, mirror-descent method, Frank-Wolf method, ALM/ADMM method, interior trust-region method for non-convex optimization, distributionally robust optimization, online linear programming, semidefinite programming for sensor-network localization, and infeasibility detection for nonlinear optimization.
New feature
The 5th edition of this classic textbook covers the central concepts of practical optimization techniques, with an emphasis on methods that are both state-of-the-art and popular. One major insight is the connection between the purely analytical character of an optimization problem and the behavior of algorithms used to solve that problem. End-of-chapter exercises are provided for all chapters.
The material is organized into three separate parts. Part I offers a self-contained introduction to linear programming. The presentation in this part is fairly conventional, covering the main elements of the underlying theory of linear programming, many of the most effective numerical algorithms, and many of its important special applications. Part II, which is independent of Part I, covers the theory of unconstrained optimization, including both derivations of the appropriate optimality conditions and an introduction to basic algorithms. This part of the book explores the general properties of algorithms and defines various notions of convergence. In turn, Part III extends the concepts developed in the second part to constrained optimization problems. Except for a few isolated sections, this part is also independent of Part I. As such, Parts II and III can easily be used without reading Part I and, in fact, the book has been used in this way at many universities.
New to this edition are popular topics in data science and machine learning, such as the Markov Decision Process, Farkas’ lemma, convergence speed analysis, duality theories and applications, various first-order methods, stochastic gradient method, mirror-descent method, Frank-Wolf method, ALM/ADMM method, interior trust-region method for non-convex optimization, distributionally robust optimization, online linear programming, semidefinite programming for sensor-network localization, and infeasibility detection for nonlinear optimization.
목차