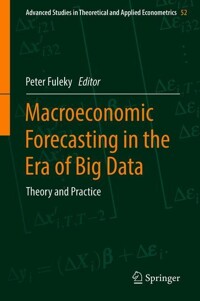
단행본2023년 BEST 30
Macroeconomic Forecasting in the Era of Big Data: Theory and Practice
- 발행사항
- Cham : Springer, 2019
- 형태사항
- xiii, 719p. : illustrations (black and white, and colour) ; 25cm
- 서지주기
- Includes bibliographical references
소장정보
위치 | 등록번호 | 청구기호 / 출력 | 상태 | 반납예정일 |
---|---|---|---|---|
지금 이용 불가 (1) | ||||
자료실 | E207367 | 대출중 | 2025.06.02 |
지금 이용 불가 (1)
- 등록번호
- E207367
- 상태/반납예정일
- 대출중
- 2025.06.02
- 위치/청구기호(출력)
- 자료실
책 소개
This book surveys big data tools used in macroeconomic forecasting and addresses related econometric issues, including how to capture dynamic relationships among variables; how to select parsimonious models; how to deal with model uncertainty, instability, non-stationarity, and mixed frequency data; and how to evaluate forecasts, among others. Each chapter is self-contained with references, and provides solid background information, while also reviewing the latest advances in the field. Accordingly, the book offers a valuable resource for researchers, professional forecasters, and students of quantitative economics.
New feature
This book surveys big data tools used in macroeconomic forecasting and addresses related econometric issues, including how to capture dynamic relationships among variables; how to select parsimonious models; how to deal with model uncertainty, instability, non-stationarity, and mixed frequency data; and how to evaluate forecasts, among others. Each chapter is self-contained with references, and provides solid background information, while also reviewing the latest advances in the field. Accordingly, the book offers a valuable resource for researchers, professional forecasters, and students of quantitative economics.목차
Part Ⅰ. Introduction
1. Sources and Types of Big Data for Macroeconomic Forecasting
Part Ⅱ. Capturing Dynamic Relationships
2. Dynamic Factor Models
3. Factor Augmented Vector Autoregressions, Panel VARs, and Global VARs
4. Large Bayesian Vector Autoregressions
5. Volatility Forecasting in a Data Rich Environment
6. Neural Networks
Part Ⅲ. Seeking Parsimony: Penalized Time Series Regression
7. Principal Component and Static Factor Analysis
8. Subspace Methods
9. Variable Selection and Feature Screening
Part Ⅳ.Dealing with Model Uncertainty
11. Frequentist Averaging
12. Bayesian Model Averaging
13. Bootstrap Aggregating and Random Forest
14. Boosting
15. Density Forecasting
16. Forecast Evaluation
Part Ⅴ. Further Issues
17. Unit Roots and Cointegration
18. Turning Points and Classification
19. Robust Methods for High-dimensional Regression and Covariance Matrix Estimation
20. Frequency Domain
21. Hierarchical Forecasting